Strict priority is a transmission selection algorithm (TSA) that is specified in the IEEE 802.1Q-2005 standard. This standard is part of the framework for the IEEE 802.1 Data Center Bridging (DCB) interface.
- Car Insurance Named Driver Algorithms. 9 February at 11:15AM in Insurance & Life Assurance. 14 replies 136 views Jd1399 Forumite. MoneySaving Newbie.
- According to Uber, fraud occurs if, for example, a driver rejects a customer, or if a driver logs out strategically in order to increase fares. Uber would never have given the drivers an explanation for the dismissal. Nor could they object. Under European privacy law, algorithms are not allowed to determine whether or not an employee should be.
With Auto-Dispatching enabled, the system will automatically assign rides to drivers for a given amount of time. You are able to determine the algorithm that determines how those drivers are selected. This is called the 'Driver Selection Algorithm', and there are 3 possible settings:
- Closest available drivers: This means that the system will choose drivers based on their location and current schedule to choose a driver who is close to the pick-up location and does not have a ride assigned during that time. The system uses a combination of distance and availability factors to make this decision, and it will assign drivers in order based on this ranking.
- Favorite drivers, followed by closest available: This means that the first drivers chosen for a ride will always be the customer's favorite drivers. Drivers will be chosen in the same order as they appear on the customer's profile. You can manage and rearrange this list on a customer's profile at any time. If the favorited drivers do not accept the ride, the system will then auto-dispatch based on the closest available driver preference described above.
- Favorite drivers only: This means that auto-dispatch will only assign this ride to the customer's favorite drivers. If the favorited drivers do not accept the ride, auto-dispatch will stop.
Example:
Let's say that you operate a fleet of 10 cars, and have your Driver Selection Algorithm set to 'Favorite drivers, followed by closest available'. A customer with 2 favorite drivers books a ride, which initiates auto-dispatch. The first favorite driver is selected, given a certain amount of time to accept, and then sent to the second favorite driver. If both drivers decline the trip, the ride will then be auto-dispatched to each of the 8 other drivers in order of their distance and availability.

Companies are increasingly using algorithms to manage their remote workforces. Called “algorithmic management,” this approach has been most widely adopted in gig economy companies. For example, ride-hailing company Uber substantially increases its efficiency by managing some three million workers with an app that instructs drivers which passengers to pick up and which route to take.
Being managed in this way offers some benefit to self-employed workers as well: for example, Uber drivers are free to decide when and for how long they would like to work and which area they would like to serve. However, our research reveals that algorithmic management is also frustrating to workers, and their resentment can lead them to behave subversively with the potential to cause real harm to their companies. Our research also suggests some ways that companies can mitigate these concerns while still taking advantage of the benefits of management by algorithm.
Together with Lior Zalmanson (Tel Aviv University) and Robert W. Gregory (University of Virginia), we conducted a multi-method study of Uber drivers in New York and London. We collected data by informally and formally interviewing 34 drivers, observing drivers in action, analyzing more than 1,000 online forum posts, and reviewing media coverage of Uber in several waves between December 2015 and September 2018.
We found that Uber drivers have three areas of consistent complaints about working “for” algorithms, concerns that we’ve also seen in other companies using algorithmic management:
Constant surveillance. As soon as they log onto the Uber app, drivers are watched and scrutinized by the platform’s algorithms; the app tracks their GPS location, speed, and acceptance rate of customer requests. It instructs them which riders to pick up where, and how to get to the riders’ destinations. If the drivers diverge from the app’s instructions they can be penalized or even banned from the platform. Regardless of whether the attention comes through an app or in person, we know that scrutiny of work can reduce productivity.
From our study we found that drivers found performance evaluations in the form of customer ratings particularly frustrating. We believe this may be because they amplify Uber drivers’ negative feelings about constant surveillance with an additional layer of technology-mediated attention.
Little transparency. While the app is learning a lot about them, Uber drivers find it frustrating how little they know about the app. They find the lack of transparency of the underlying logic of the complex algorithms frustrating, believing it to be an unfair system which manipulates them subtly without their knowledge or consent. (Indeed, Uber has previously admitted to drawing on insights from behavioral science to nudge drivers to work longer hours).
Uber drivers, as well as other gig economy workers such as courier and delivery workers at Postmates and Deliveroo, are demanding more transparency about the allocation of jobs, the compilation of their ratings, and their payment structure. However, companies such as Uber argue they can’t reveal the secret recipe of their algorithms to competitors. Furthermore, recent advances in AI and machine learning mean that algorithms can now learn and dynamically adjust to any given environment, allowing for the automation of more sophisticated tasks (such as managing the workforce). But the more sophisticated these algorithms get, the more opaque they are, even to their creators.
Dehumanization. Drivers at Uber report feeling equally lonely, isolated, and dehumanized. They don’t have colleagues to socialize with or a team or community to be part of. They lack the opportunity to build a personal relationship with a supervisor. Those on crowd-work platforms like Amazon Mechanical Turk have raised similar complaints as they conduct “micro-tasks” such as classifying content or participating in surveys.

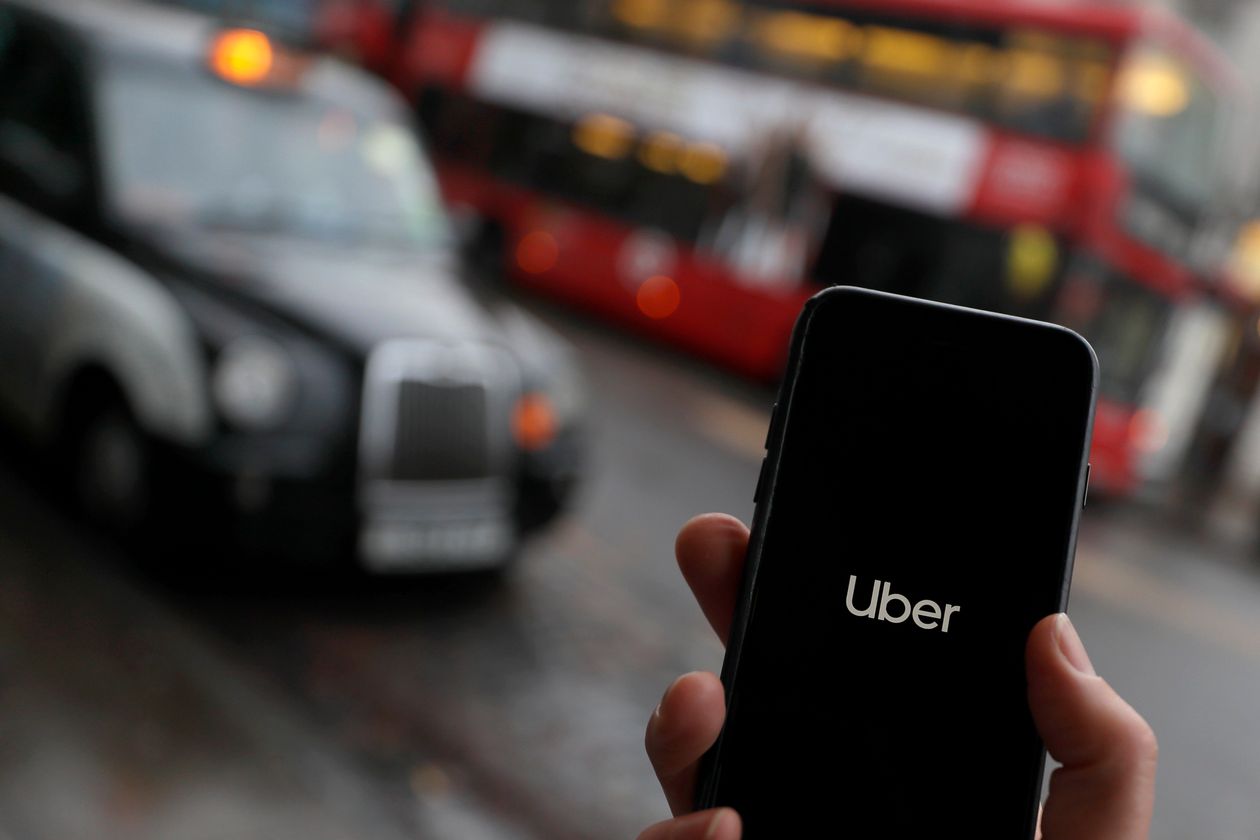
Drivers have responded to their various frustrations with these algorithms by identifying clever ways to work around them. For instance, one driver in the Uberpeople.net forum wrote: “Play the system, don’t let it play you. We all know that these companies like to offer better incentives to drivers that miss some time. So, drive Uber for one week, Juno next, Lyft third and etc. I switch between Uber/Juno weekly.”

They are also angry enough — and feel disempowered enough — that they are finding creative ways to make their displeasure known; for instance, drivers are gaming the system by artificially causing surge pricing. They are also getting political; especially in the gig economy, drivers of ride-hailing services and couriers seek to compensate for the social isolation they experience in their every-day routine by actively engaging in online communities, but companies themselves aren’t involved in those platforms. Instead, more-adversarial union-type organizations have sprung up as drivers or couriers look to support each other, such as Seattle-based workers’ rights organization Working Washington, which drew together couriers delivering for Postmates, DoorDash, and other on-demand services.
Driver's License Number Algorithm
In order to address these challenges and mitigate their risks to the business — and, not least, to implement ethical practices — we suggest that companies who manage all or part of their workforce through algorithms:
- Share information. In theory, algorithmic management can increase transparency, since even learning algorithms that are used to manage workers reflect a set of rules and procedures that comply with the strategic goals of upper management. It may not be possible to share the algorithm itself with workers, but company leadership can and should share with them the data and goals that informed it.
- Invite feedback. To counterbalance the unidirectional commands that the algorithm hands down to drivers, companies should find ways to democratically include them in decision-making, for example by involving them into committees or councils that discuss and negotiate work related internal regulations. Getting workers actively involved in discussions about the design of algorithm-driven systems would do much to build more engaged and supportive workforces.
- Build in human contact. People need people. Organizations should develop formal, supportive communities where workers feel like members and can make social connections. Adding a human element to the way people are managed will help workers feel less like they are being treated as machines. For instance, some of the drivers in our study spoke fondly of New York ride-hailing firm Juno (acquired by Gett in 2017), which, early in its existence, employed an extensive human customer support system that eagerly helped drivers with questions or problems.
- Build trust. Implementing benefits that improve worker’s welfare, such as providing financial support in case of illness, or better sick pay or maternity leave, may be a first step to humanizing the company and mitigating the anger of employees who are managed by faceless algorithms.
Uber Algorithm Drivers
Regulators across the globe are already seeking to implement regulations around some of these recommendations in order to benefit an algorithmically managed workforce. For instance, in 2017, it was ruled that Uber will need to pay UK-based drivers a minimum wage, and provide sick and holiday pay. Given the rapid pace of technological progress, and the tempting economic benefits for companies, we believe that algorithmic management will become more common in the coming years. As more companies manage their labor force in this way — and as they incur the anger of the workforce that makes their core offerings possible — it becomes that much more incumbent upon them to take some of these steps on their own.
